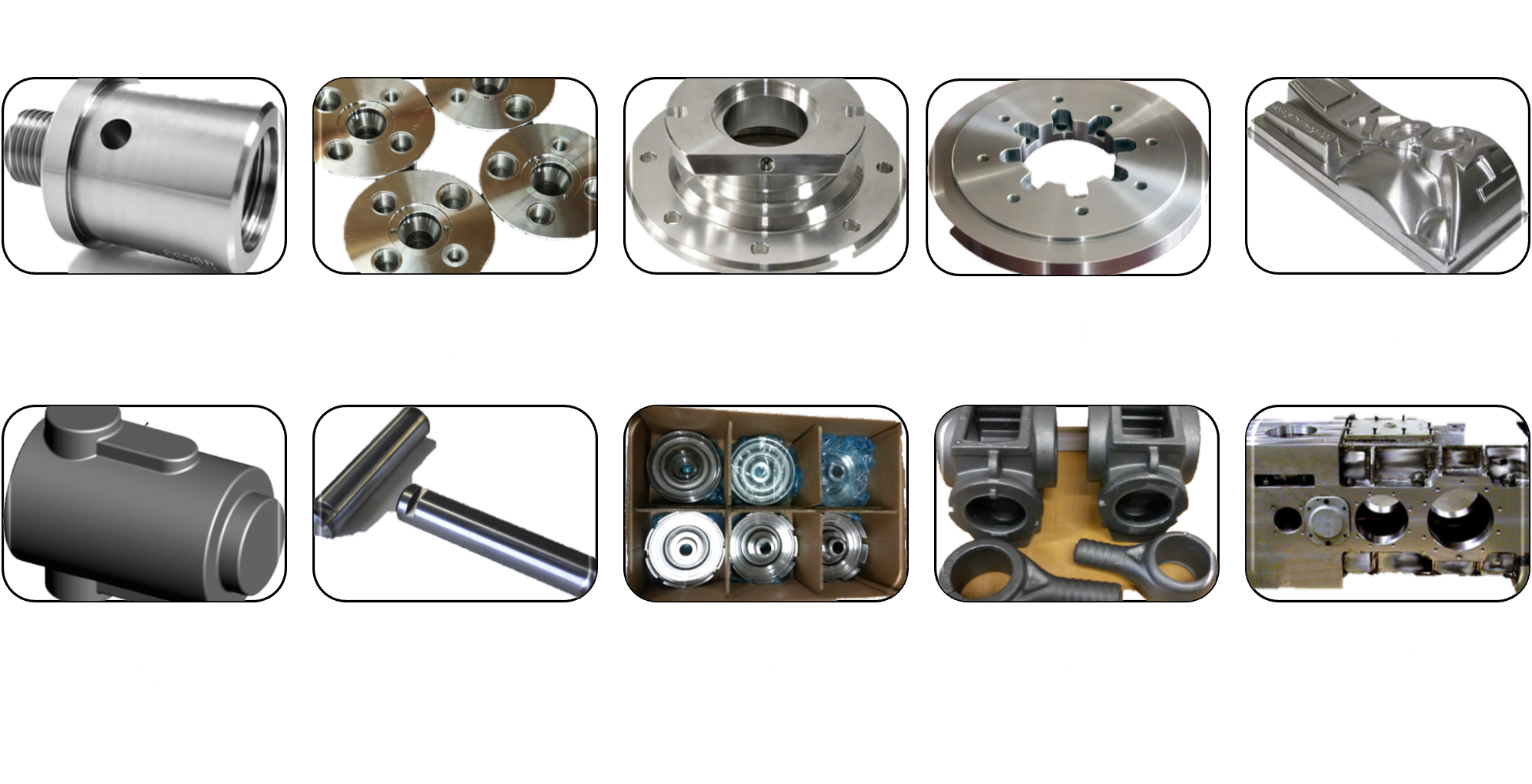
Vision Inspection of Rotors for rotary screw air compressors
Using Company Proprietary Image Signature Method
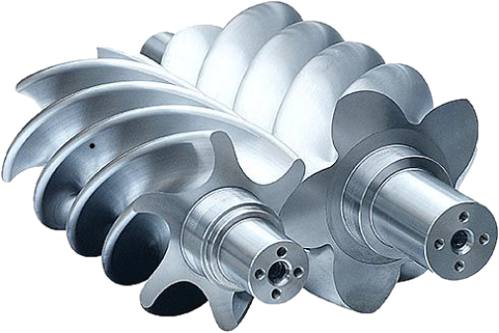
Rotors of rotary screw type air compressors have complex geometry. When they come out of grinding machines, various pores happen to appear on the surface. Some of these pores are big enough to consider as defects. These pores have arbitrary shapes and geometries and present a challenge to detect using conventional machine vision systems if they lie in shadowed areas caused by the geometry or at edges.
IOIMACHINES demonstrated a solution for this problem. The solution is based on advanced feature detection combined with powerful machine learning techniques. The solution is tested on a collection of images of both good and bad rotors of different sizes and geometries. Test results show that we can achieve 100% accuracy in detecting all types of defects over a predefined size. The solution distinguishes between types of defects and the system learned to distinguish real defects from oil films and droplets as well as air bubbles in dirty oil, shades and shavings.
In the solution, new types of defects and artifacts are learned quickly with minor intervention from qualified operators.
Main advantages seen by the customer are:
- No reprogramming or re-engineering is required
- Zero downtime
- Zero escapements
- Seamless solution for different rotors of different shapes and sizes
- Minimum intervention from operators
The solution is provided for all types of grinded objects
Wind Energy Rotor Blade inspection
Detecting defects on the inner surface of the shell for a wind energy rotor balde
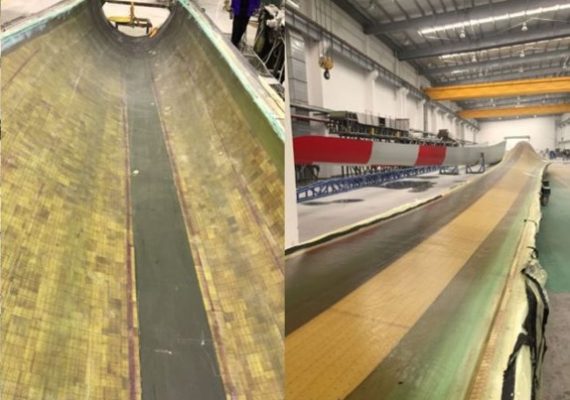
A feasibility analysis of implementing a Quality Inspection system for wind turbine blade shells using Computer Vision.
The study responds to Project objective to study the technical feasibility to use Computer Vision and Artificial Intelligence for inspections. Scope for analysis has been shell inspection after infusion and debagging according to given standards.
Fieldwork has been done on blade types at a production Plant. A large number of images has been taken and analysed. Pictures correspond basically to standard structures and also to defects like LAV, LDL, foreign objects, etc. present in production shells.
Image processing algorithms tested through the feasibility study proved efficient in locating and identifying defects on the shell surface. Studied image analysis techniques show that different defects change the image contents in different ways which show to be detectable. However due to the variety of basic surface structures and significant variation in the appearance of the defects on these, it is concluded that these conventional image processing algorithms are not sufficient to detect all types of defects and classify them. Therefore, the feasibility study concludes that there is a need to adapt a machine learning approach. In the approach, the system learns from experienced operators to classify and categorize new types and variations of defects, as well as new variations of basic surface structures. The system accumulates learned knowledge and the need for teaching the system declines rapidly as function of number of correct training sessions. The machine-learning scheme provides a robust method to detect all types of defects invariant to their scale and rotation as well as light variations and variations of structural backgrounds even in the presence of normal artifacts on the shell surface.